Creating an accessible future for AI.
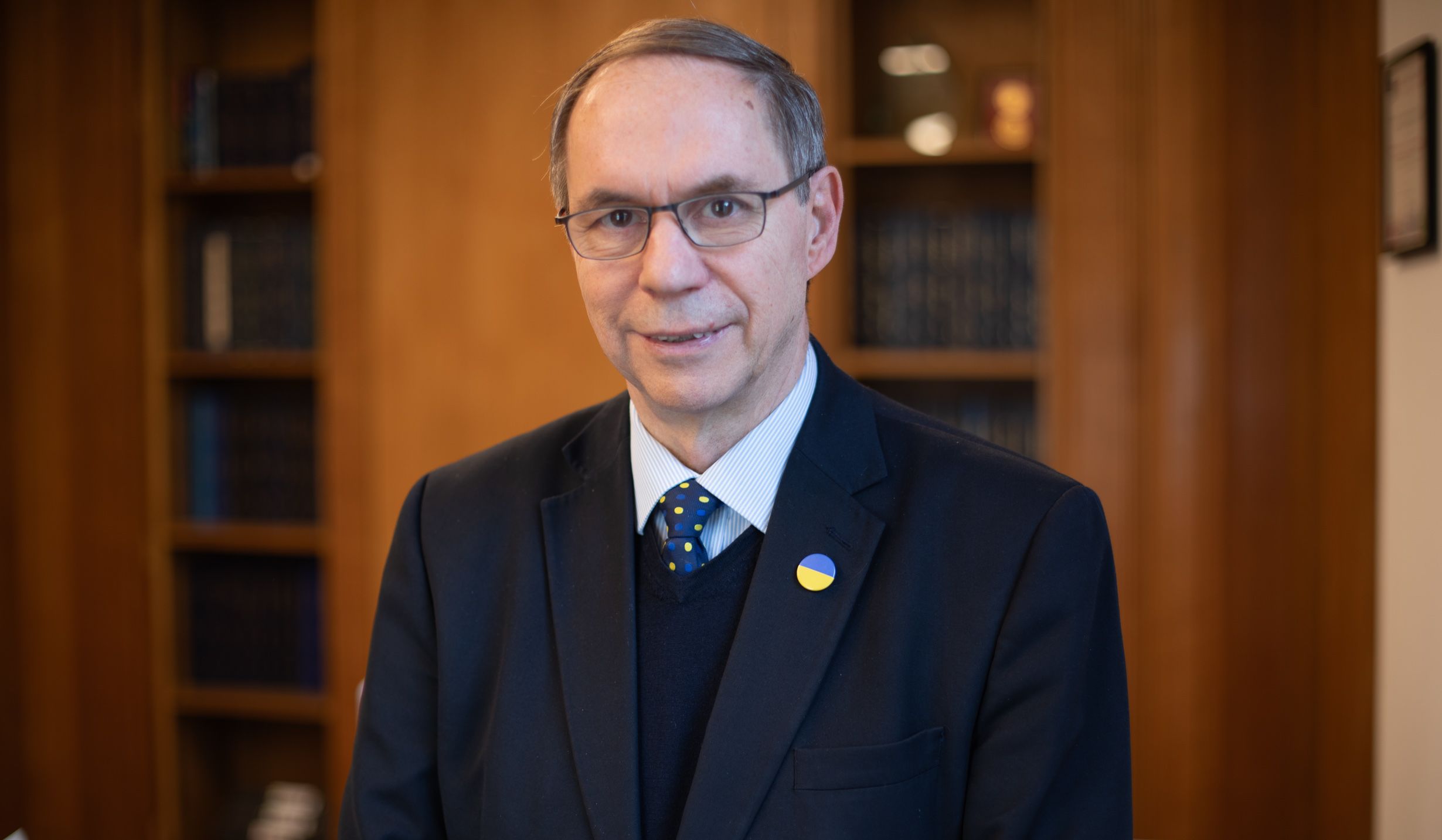
In 40 years at Oxford, Lionel Tarassenko has moved 100 yards.
The Professor of Engineering Science in the Department of Engineering Science first came to Oxford as an undergraduate student in the late 1970s, studying Engineering Science and later, a DPhil in Medical Electronics at Keble College.
With a nuclear physicist father, engineering was a ‘natural fit,’ he says.
Today, he is also Founding President of Reuben College, established in 2019 as the University’s 39th college, whose entrance is 100 yards from Keble’s entrance.
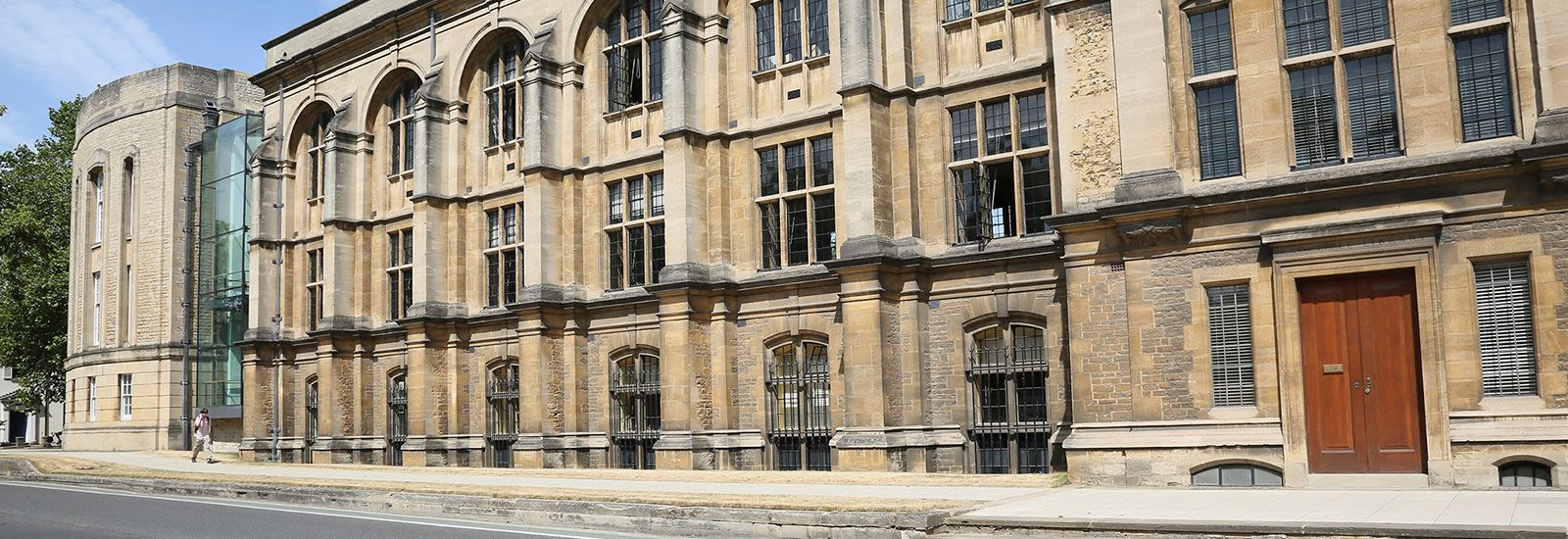
Reuben College
Reuben College
Having completed his undergraduate studies and being interested in the emerging telecoms field, Professor Tarassenko worked in industry for two years at Racal Electronics, where he experienced first-hand the emergence of a world-changing technology, the mobile phone.
As a young engineer working on queuing theory, he was responsible for estimating how many calls there could be in each cell of the Racal-Vodafone mobile network. The estimates were completely wrong, however, because they were based on a projected number of 50,000 subscribers for the whole of the UK.
Returning to Oxford in the 1980s first for his DPhil and then as a Tutorial Fellow at St Hugh’s, he began investigating neural networks, an early name for what would come to be known as machine learning.
‘There was a first explosion of research from about the mid-80s,’ he says, ‘I was interested in neuroscience and therefore in any kind of algorithm based, perhaps even loosely, on how the brain computed.’
‘A lot of people were very sceptical about the subject, saying I was probably wasting my time. I occasionally remind them of this, when they discuss their latest machine learning research with me.’
This research was not without sceptics; the early field of machine learning had found itself frozen in previous decades.
Yet, Professor Tarassenko points out, the fundamentals have not changed, with even the most advanced neural networks seen in today’s generative AI based on the same building blocks and learning algorithms he was working with at the time.
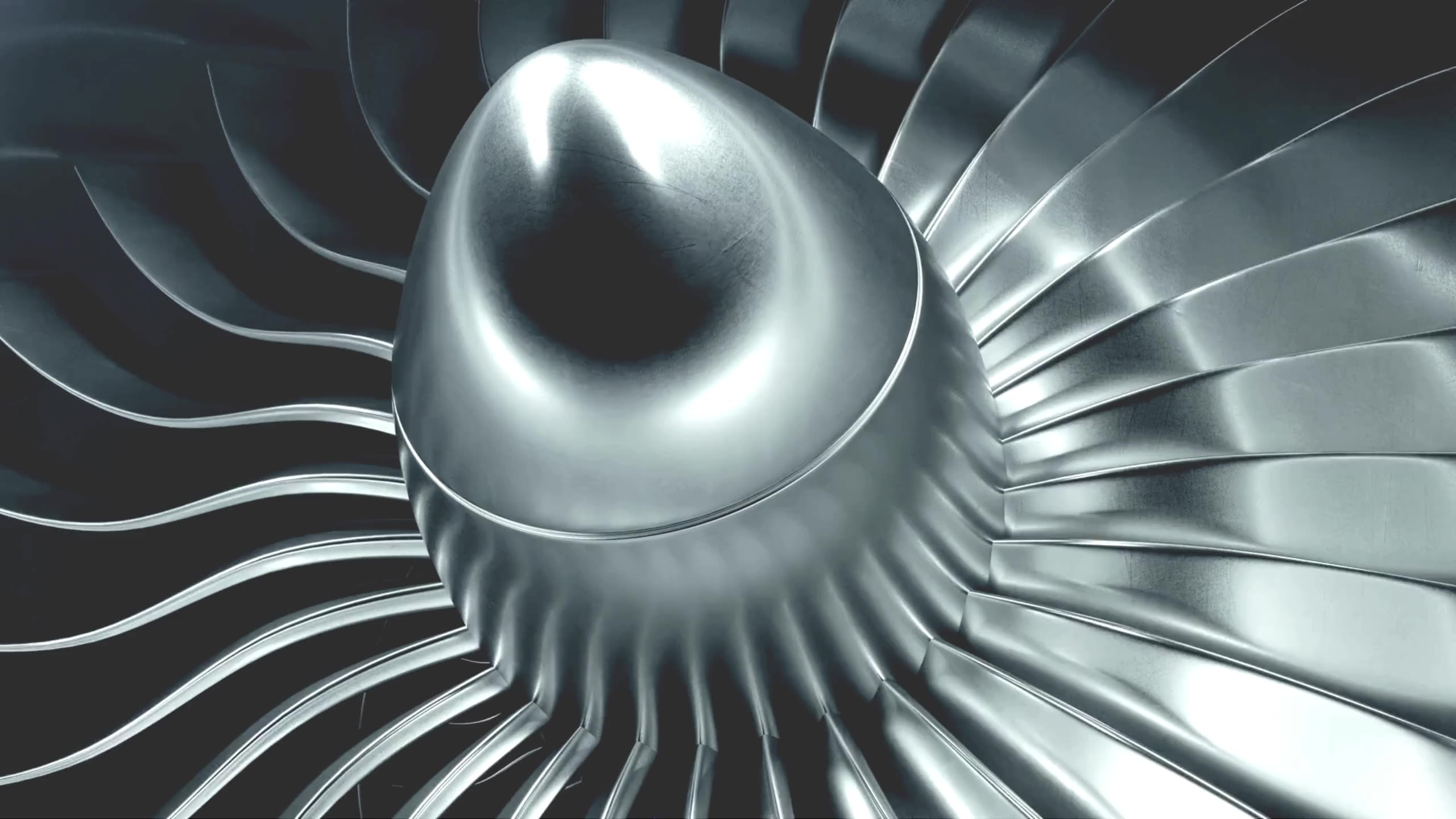
Professor Tarassenko has always been interested in the real-world applications of machine learning.
As one of the first researchers to see the potential of learning from data, he received a British Computer Society Medal in 1996 for his work on neural network analysis of sleep disorders, the first given for a machine learning application.
At the time, the scale that these applications would come to have ‘could not have been imagined,’ he says.
‘It now sounds obvious because everybody's using machine learning algorithms to obtain wonderful results in biomedical image analysis, processing CT scans, for example.’
His work on sleep disorders using neural networks to classify different types of sleep is as relevant today as it was in the mid-90’s; with his work on analogue circuits for implementing neural networks, he now sees people returning to this way of thinking, looking for more efficient ways to train very large generative AI models such as ChatGPT which require a ‘huge amount of energy.’
‘Work I was doing 30 years ago is coming back into play, which is very interesting to see. And you do get these cycles in a field of research if you stay long enough in the field.’
Professor Tarassenko’s health monitoring applications of AI have not been limited to the medical field.
He further developed his research to investigate jet-engine health, working with Rolls-Royce to develop early detection systems for abnormal patterns in engine vibrations, temperatures and pressures, to anticipate maintenance needs.
His work was awarded the Rolls-Royce Chairman’s Award for Technical Innovation in 2001 and the Sir Henry Royce High Value Patent Award in 2008.
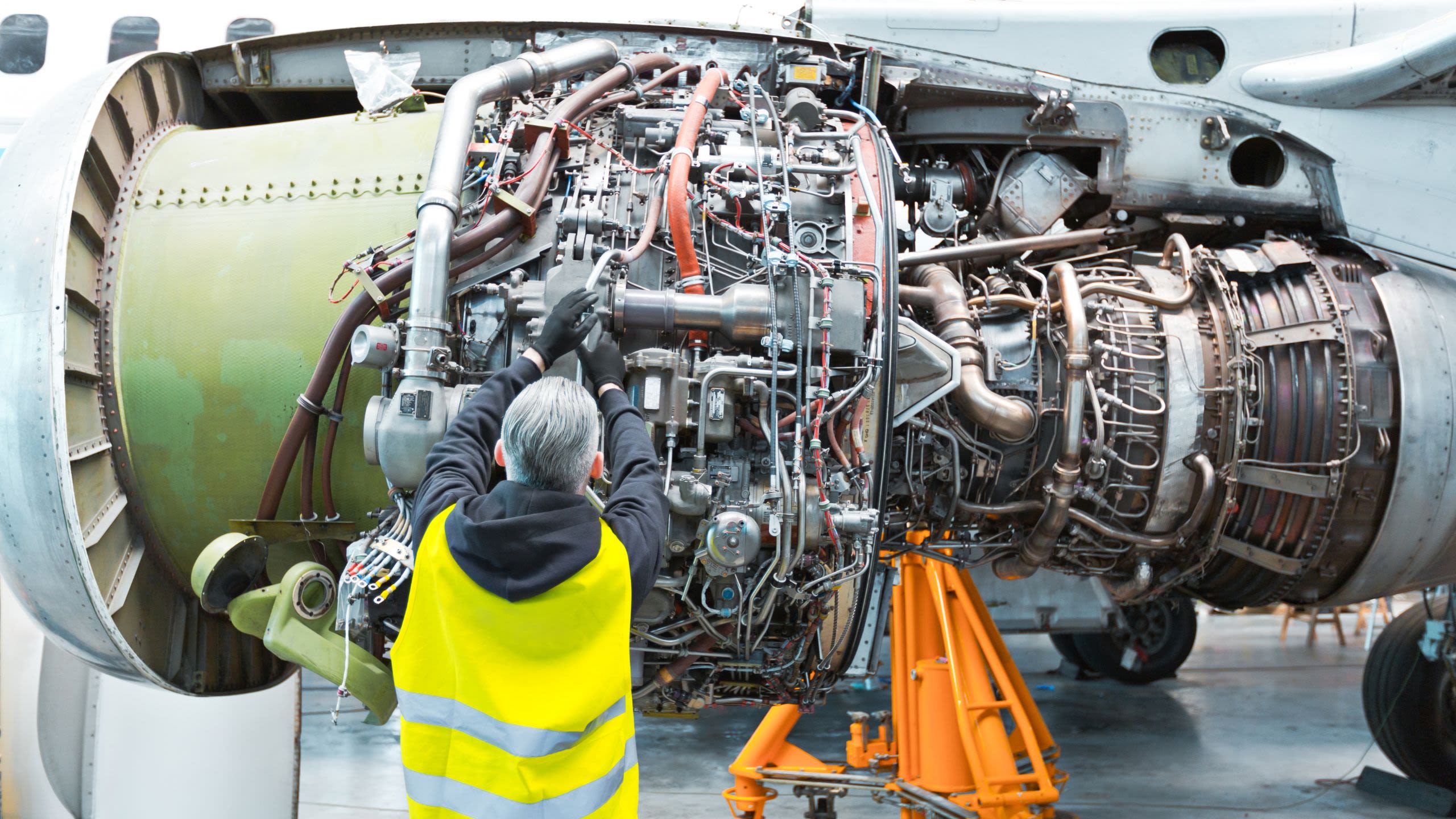
The safety critical systems developed during his time with Rolls Royce provided the basis for another of Professor Tarassenko’s ideas for developing digital healthcare.
Returning to medical applications, he developed patient monitoring systems including wearables for in-hospital patients and monitoring of blood glucose and blood pressure in high-risk pregnant women.
Key to all these developments, he is keen to point out, is access to high-quality data. ‘It's essential to have the right databases to ask the right questions and develop new applications,’ he says.
‘In Oxford, in the medical sciences, we've got amazing databases like UK Biobank, like The Million Women cohort, the [China] Kadoorie Biobank. So we’ve got this explosion of fantastic databases.’
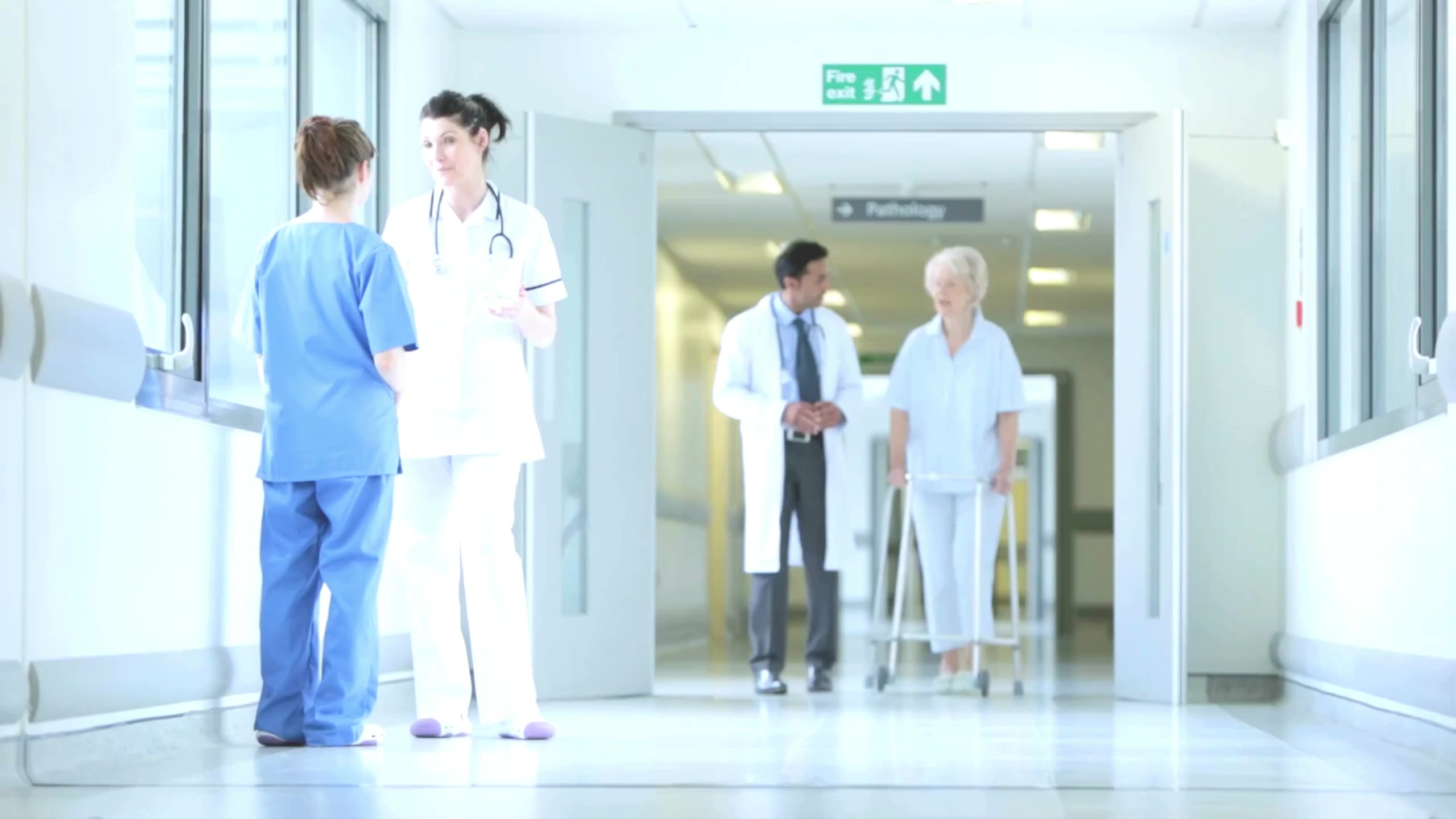
In a ‘much-hyped’ field, Professor Tarassenko wants an understanding of AI to be accessible to students.
At Reuben College, he offers regular talks on AI and machine learning, with an emphasis on explaining to a general audience ‘how it works and what its capabilities are.’
‘Our students may be studying philosophy, geography, medicine or public policy. I want AI to be accessible to all, and at the start of the year I gave a kind of TED Talk to explain what lies behind these large language models, which are tremendously powerful.’
A recent presentation given by Professor Tarassenko on Chat GPT.
As part of the ‘Dining with Dinosaurs’, so named because of their original location in the adjacent Natural History Museum, these talks reflect an underlying ethos of Reuben, where students from different disciplines are brought together to interact and discuss topics such as AI, climate change or the latest medical advances.
‘That’s the kind of thing we do at Reuben, deliberately think about multi-disciplinarity, and not wait for serendipity.’
‘We are really focused on promoting and enhancing multi-disciplinary interactions amongst fellows and students,’ he says. ‘We set out to have four founding themes, which are AI & machine learning, cellular life, environmental change, and ethics & values.’
This cross-disciplinary community of researchers has become home to 10 (soon to be 25) AI in Science Research Fellows, with the recent awarding of The Eric and Wendy Schmidt AI in Science Postdoctoral Fellowships to the University.
Professor Tarassenko says the new Research Fellows are a welcome addition to Oxford’s AI expertise in STEM departments, where they will be placed to make developments across a wide range of fields.
‘They will join a thriving community of academics and graduate students working in related fields. Reuben College will be the place where they can gather together to meet outside of their Departments, which are scattered across the University, and join not only in the intellectual life of the College but also its social activities through membership of the Graduate Common Room.’
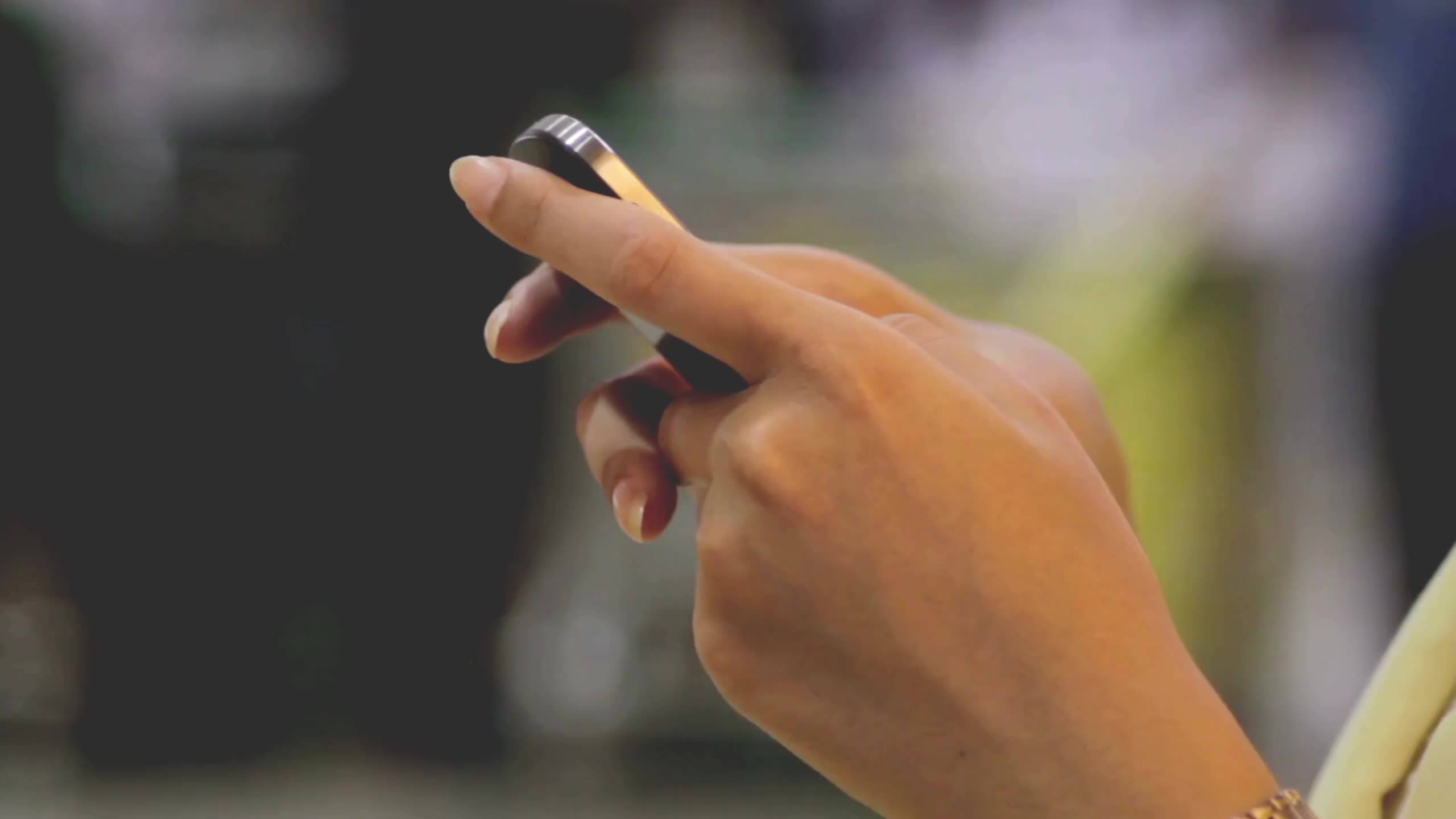
Oxford’s expertise in the AI space has attracted the attention of 'Big Tech' companies.
This can, according to Professor Tarassenko, be attributed both to the explosion of new AI research as well as the continued work of leading researchers in this space, such as his colleague Professor Andrew Zisserman with whom he shared an office early in their respective careers; Professor Zisserman now holds a joint appointment with Google DeepMind.
‘There’s a lot of academic-industry collaboration in Oxford, with ‘big tech’ companies collaborating with Oxford researchers, driven by the explosion of applications in computer vision and robotics, for example.’
‘When all 100 Schmidt AI in Science Postdoctoral Fellows are here, this will probably be the greatest concentration of post-doc researchers in AI anywhere in the UK,’ he says. ‘We've got this amazing intellectual resource in Oxford.’
When looking to the future of the field, he wears the hat of Senior Director of Oxford University Innovation, a role he holds having founded four University spin-out companies (and he is thinking of spinning out his lab’s research on generative AI for sleep monitoring into a fifth company...)
Despite the close associations with 'Big Tech', there is more to be done with the ‘amazing resources’ for spinning out companies available to Oxford’s researchers, he notes.
‘I’m hoping to raise funds to generate new AI spin-outs from the fantastic work being done in computer science, mathematics, statistics, engineering, the Big Data Institute, etc.’
‘And the key thing is, in order to be able to compete in that field, you need large amounts of money because you need to move fast. You can't wait 10-15 years, like life sciences companies developing a new drug. To be successful as an AI spin-out, you have to get there in two or three years.’
More #OxfordAI experts
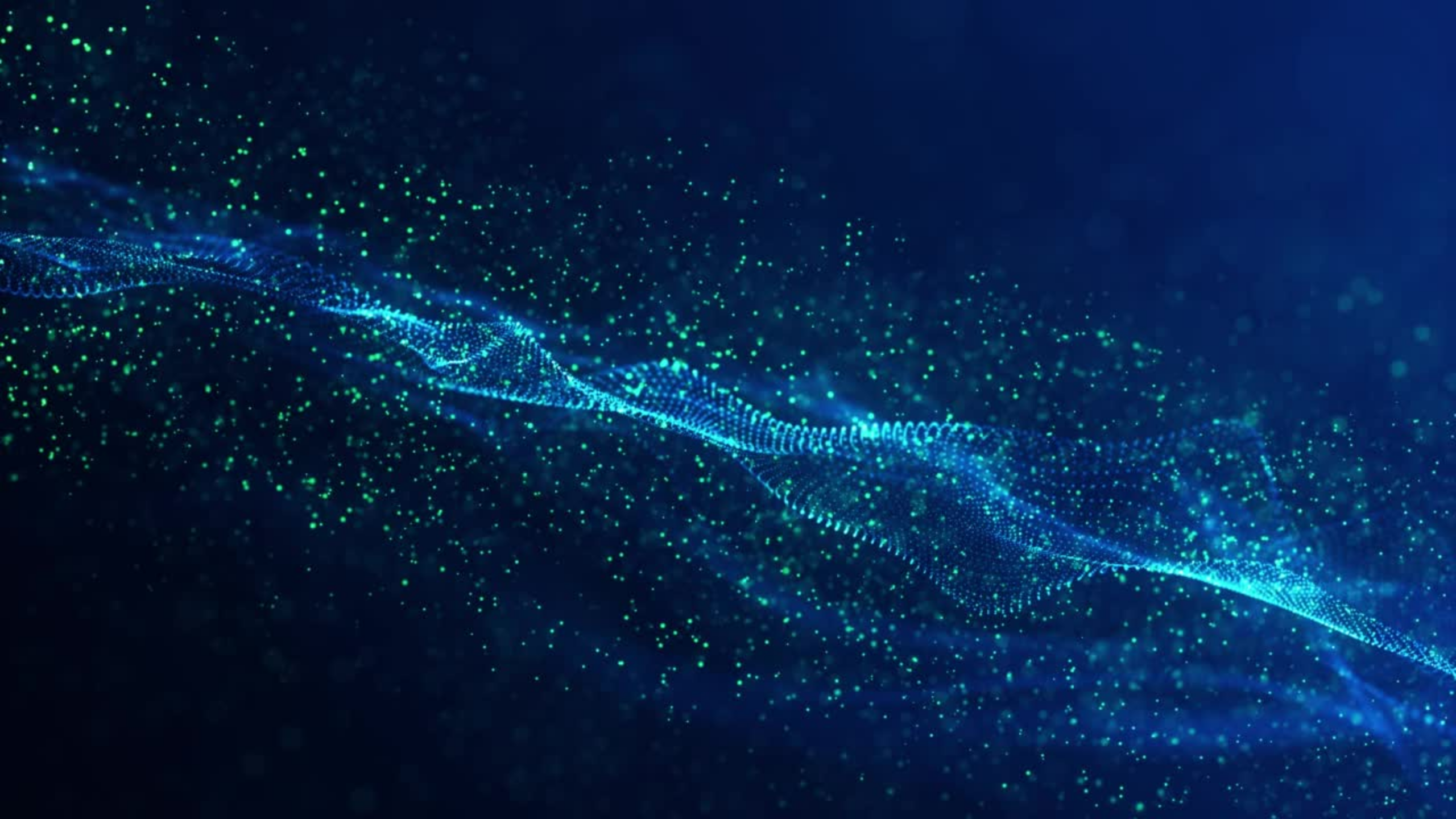